The Content Trading Desk
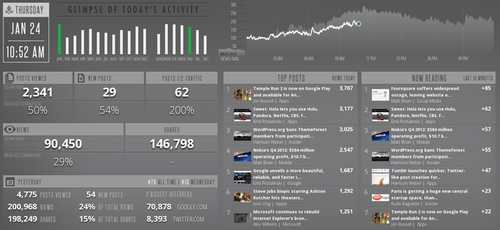
My first job out of college was as a software engineer for Morgan Stanley. This 50,000 employee firm employed tens of thousands of software engineers. Though the most important employees were the traders, it was clear that software ran the place.
For anyone who has worked on Wall Street in the last couple of decades, this is no surprise. It may be surprising to people from outside the industry, who perhaps still associate Wall Street with traders wearing funny jackets calling out orders across a busy NYSE trading floor. That still goes on (though, probably not for long). Most of the trading activity on Wall Street is heavily computerized. Orders are placed and fulfilled mostly by machines.
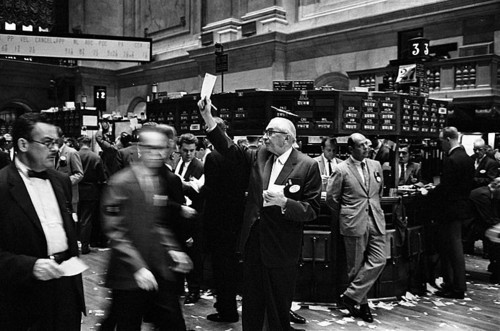
Once the markets went digital, data analysis became a much more rigorous discipline on Wall Street. Whereas before, a trader’s primary edge was being personally connected to the most important players (think Gordon Gecko), today’s traders seek edge in systematic prediction models. In other words, other machines analyze the orders being placed and fulfilled on the market, and yet others try to detect patterns or make predictions based on all of this data.
One interesting aspect of Wall Street is that what most people think of as “the market” is nothing more than a transaction log for all the buy and sell orders happening minute-by-minute, second-by-second (and lately, microsecond-by-microsecond). Because analyzing all of this data in real-time is a tough processing challenge, Wall Street has opted for a hybrid human-machine combination for everyday decision-making.
Some small divisions do 100% automated trading (usually called “algorithmic trading” or “high-frequency trading”), but most outfits rely on experienced human beings who are empowered by (not replaced by) technology. Data visualization plays a critical role in overcoming the gap — putting lots of data in front of a skilled pair of eyeballs and brain. Modern traders also use technology to provide relevant contextual information, to communicate with peers, and to perform research.
This is perhaps best embodied by Wall Street’s premier technology status symbol, the Bloomberg Terminal.
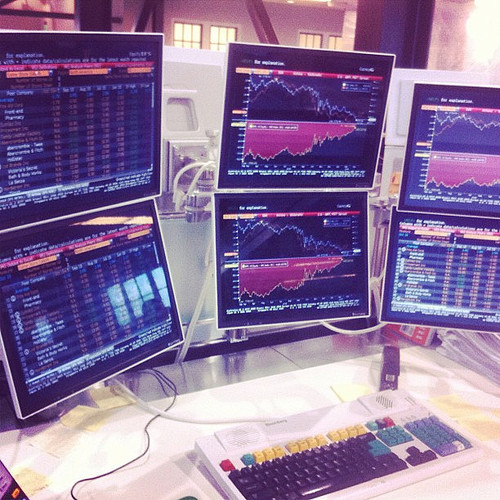
For the last year, as I worked with media companies implementing Parse.ly technology across their organizations, I started to see an interesting parallel between Finance and Media. Like in Finance, the world of Media is being turned upside down by the Digitization of Everything. The front-line employees who live in this industry have to establish new competencies that are digitally native. Unsurprisingly, now that content consumption has become a digital experience, it can be more directly tracked and measured — and this data can create important real-time feedback loops for these employees.
One other important parallel is the shape of the data. Content measurement is similar to market measurement: fast-moving time series ticks, loaded with important metadata, full of peaks and troughs. Further, millions of correlations exist between trends in the content ecosystem. Traditional web analytics products (like Google Analytics and Omniture) are the Wall Street equivalent of the raw market feed.
Media is also deeply unlike Finance in a number of important ways.
First, the monetization stories are dramatically different. In Finance, monetization is staggeringly straightforward. One might even say Finance simply is monetization, in its purest form. As a result, investment in technology is easy to justify. Bloomberg’s $2k/month license per seat is easy to justify as many large firms can make millions per month off each of their traders. In Media, monetization is a complicated little rabbit hole of its own. People aren’t typically buying content; they are usually consuming it for free. Content creators and editors are viewed as the cost center, not the profit center, of these organizations. Attention is indeed monetizable, whether through advertising (short-term) or subscriptions (long-term), but the relationship between traffic and revenue is not direct.
Second, actionability is still hazily defined for this industry. Editors and writers can typically control the links and content that appear on their site, but not much else. The search era brought in some new knobs (keywords, long-tail queries) and the social era brought in a few more (tweets, shares, virality). But for the most part, data has only provided a rear-view mirror perspective. Traders, by contrast, are eminently action-oriented: they need to decide whether to buy or to sell. Historical data might help an editor or writer learn from success or failure, but how could it lead to their acting in a predictive manner?
The answer is the network.
We’ve made a business by identifying that content creators need technology help in making everyday decisions. Neatly organized historical and real-time visualizations of their traffic data has allowed them to create order from chaos. On any given top content website, there are millions of URLs and billions of content interactions (pageviews, links, social shares, search queries) across a slew of platforms (web, phone, tablet). With content lifecycle measured in hours rather than days, opportunities pass by quickly. The tools we’ve built have helped bridge the gap and create the “human-technology” hybrid editor.
But I’m most excited about the fact that what we’ve been building is actually the content equivalent of the NYSE itself (or, perhaps more accurately, NASDAQ).
Across Parse.ly’s entire network of sites, we have grouped traffic signals by publication, topic, tag, section, referrer, and lifecycle stage. By providing the network view back to each of our publishers (in aggregated and anonymized form, to protect each individual publisher’s privacy), we can let the entire media industry benefit by turning every editor and writer into a content trader.
Imagine: a content trading desk. Content suggestions stream on the screen. Based on topical trends, editors can make “buy” or “sell” decisions. “Buy” means invest in content creation / licensing and grant it appropriate seed traffic. “Sell” means starve it of attention and let it disappear from view.
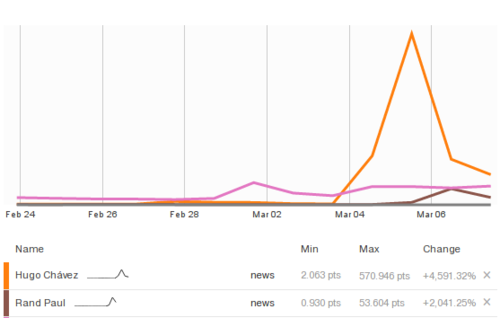
Operating in this data-driven way won’t mean instantaneous profits for online media. It may even create some strange echo-chamber effects, as has certainly happened in our financial markets. What’s more, some editors will make the wrong investments, despite being armed with the right data. Well-armed traders are also wrong much of the time. Some contrarians may even make a business out of willfully ignoring the conventional interpretations.
Yet, once this data is available, I think it will be hard to justify “flying blind” any longer.
—Andrew Montalenti, Co-Founder and CTO
Reposted (with permission) from Medium