How Recommendations Shape Reader Preferences
Digital media has empowered readers like never before. With a seemingly endless supply of content and the newfound ability to contribute via user-generated content and social media, readers are now calling the shots, and media organizations can only chase and adjust to user preferences in an attempt to get a leg up on their competition.
This is the prevailing wisdom of the time anyway, but color Dr. James Webster skeptical of all that. In his award-winning book The Marketplace of Attention: How Audiences Take Shape in a Digital Age, Webster presents evidence of how existing media structures, specifically recommendation algorithms and measurement biases, are cultivating a reader’s interest in topics, but not necessarily allowing readers to act on preferences they had before visiting the site.
In other words: Were readers already interested in the stories shown in recommendations, or are readers becoming interested in stories because they’ve been recommended?

Digital media organizations are assuming that they’re chasing user preferences, but what if the reality is the reverse: that publishers shape user preferences? I chatted with Webster about this chicken-and-egg question, and how else the digital sphere is shaping media.
The Readers vs. the Recommendations
Jeff Andrews: Has digital media empowered the audience or has it merely presented the illusion of power?
James Webster: In some ways it is a bit of an illusion. Readers certainly have more choices than ever before. If you take that at face value, you could say “well the users are now in charge.” I guess that’s where I part ways with them.
But in order to make sensible choices you need help — it’s what people call the paradox of choice. So you rely on recommender systems to define what you’re after. When you rely on those sorts of systems you’re vulnerable to whatever biases they have.
Andrews: The origins of people’s preferences for types or topics of web content, movies, television shows, podcasts is a big topic in your book. What are some of the factors that shape a person’s preferences?
Webster: Broadly speaking, the preferences arise in one or two ways. Exogenous preferences develop outside the media system, for example, from your social class, your education, your occupation – what some academics call your cultural capital. Less carefully considered by academics is the alternative: preferences that rise from within the media environment. So you’re there visiting websites, and you’re exposed to things that become preferences. These would be endogenous preferences, something that arises within the system.
Andrews: So given that, are the existing recommendation systems giving readers what they want or are they part of a system that’s shaping their preferences?
Webster: The recommendation engines are sometimes self-fulfilling prophecies. If you bill something as the “most-read story” it could become the most-read story because people gravitate to that. Part of the problem is that the things that determine the recommendations you see are often a black box so you don’t know what’s gone into the recipe for recommending something to you.
You’d like to think that these recommendations are always in the interest of the media user, but what is in the interest of the media user might not always be consistent with the interests of the recommendation system. As a rule they do a good job of either saying this is popular, you might want to take a look at it, or they’ll do something more refined and personalize a recommendation on the basis of whatever data they have about you.
Andrews: You say media structures and measurement biases shape reader preferences for content. Others say that user preferences are ultimately shaped by the media structure. Who’s actually pulling whose chain?
Webster: That’s the $64,000 question. Certainly the rhetoric is that the balance of power has shifted. I think that’s a misreading of the situation. I think this notion that people are finally in charge causes you to turn a blind eye to all the strategies and resources that the media — from traditional media institutions to people who create and share content — to draw your attention and push things at you to determine ultimately what it is you see or read or hear.
Causation flows in both directions, which may not be the most satisfying answer. It’s not an either/or proposition. What ultimately attracts an audience is a collaborative effort between media — which has prerogatives and ways to push things — and audiences who now do in fact have a measure of power to shape the media environment.

How Data Influences the Recommendations
Andrews: Let’s say I’m a news media website and I want to make a recommendation engine that will legitimately present something to the reader that they might want, that’s free of the structure and measurement biases. How might I approach doing that?
Webster: You’d need to think about the kind of data you have, and how you want to use that to craft the algorithm that will make recommendations.
For example, behavioral data, like pageviews or visitors, show which pages that have attracted attention. Another metric (like engaged time) will show if people spend a larger amount of time looking at one story over another.
You have these, and at least a couple of other candidates for what you might want to consider. To oversimplify for the example above, what you would have to decide is: are you going to put more weight on the size of a story’s audience or are you going to recommend stories that appear to be the most engaging?
Andrews: Could a system present multiple options? One story where the audience size was large, one where a story was well-read and another that’s of a topic the reader has enjoyed in the past?
Webster: That’s possible. You see something like that on some websites where you get the most popular, the most emailed, the most commented upon. I think that’s an option. You don’t want to offer up recommendations that are needlessly confusing, not that what you suggested is particularly confusing, but you don’t want to overwhelm people with choices if you’re offering recommendation.
How Trending Topics Influence the Media
Andrews: Are you concerned about the combination of pre-existing preferences and personalization of content recommendation isolating and fragmenting audiences to an extreme degree?
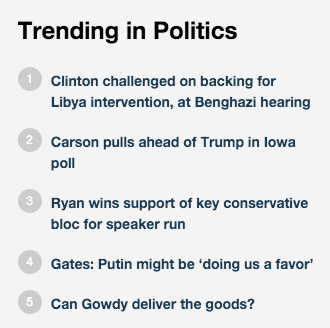
Webster: Everyone’s intuition — and this extends to a lot of theorists — is that people with certain ideological predispositions would spend a lot of time with either red media or blue media, depending on their preference. They’d basically shut out what they’re not inclined to agree with. But that just doesn’t happen for the most part.
Let’s take Fox News as an example. A very small percentage of Fox News’ audience (20 percent) accounts for an overwhelming amount (95 percent) of the time spent viewing Fox News. Other people who are Fox News viewers don’t spend a lot of time with Fox News. They all for the most part tend to be heavy media consumers. They watch a lot of everything. The idea that just because you watch a lot of Fox News that you’re living in this environment where you’re not exposed to other ideas is kind of misguided, and it isn’t supported by the evidence.
Andrews: Many news media get caught in what you call “the spiral of sameness.” One way I experience this is that every Monday my RSS feed is flooded with John Oliver recaps. How has the influx of data into the editorial process contributed to this?
Webster: In a digital environment you’re keeping an eye 24/7 on what your competitors are doing. If they cover a story, it’s very easy for you to quickly replicate that. You frame it a little bit differently but it’s the same story. And it’s constant. You don’t have the lag time you did in a print environment.
Data’s role is a little harder to know, but you have a rush to cover things that demonstrably show popularity. The more popular they are, the more you would feel under the gun to cover it or lose readers.
I’m suspicious of using things like trending topics on Twitter as a reliable indicator of what’s going on. You’re really looking at a black box. You don’t know how those trending numbers are being cooked up and how Twitter is weighing various factors. Some academics try to look at all the documentation they can find but it suggests there are a number of things baked into the numbers that wouldn’t be apparent to an ordinary person.
Do you make editorial judgments based on numbers that you don’t really understand? You think you understand them but you actually don’t.
Thinking about using data to create your own recommendation system? Check out Parse.ly’s API.