What Parse.ly Learned from Trying to Do Predictive Analytics
Whether you need to craft a landing page that will drive sales, or a blog post that will spark engagement, analytics can help you to enhance the power of your content through a better understanding of what is driving visitors to your website, and why.
In a recent Computerworld post, Parse.ly CEO Sachin Kamdar writes about the challenge, and the opportunity, of predictive analytics for digital publishers and media companies. Predictive analytics uses data to make predictions about future events. But for digital publishers, the data too often provides insight in retrospect — leaving little room for actionability.
Did you know that Parse.ly originally billed itself as a “predictive analytics platform”? Check out our vintage branding below!
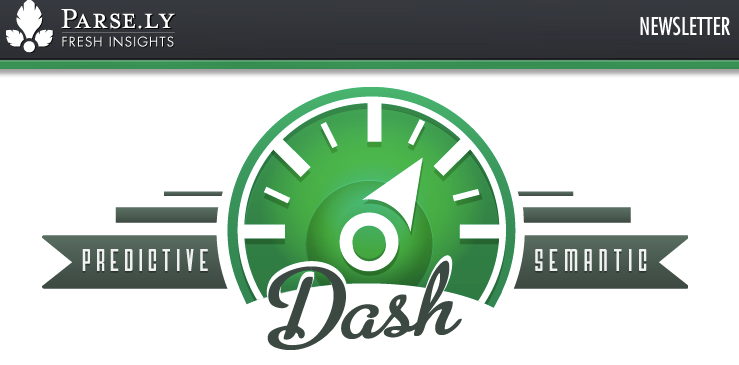
So what happened? Why don’t we bill ourselves as a predictive analytics provider today?
Perhaps one of the biggest lessons learned from early Parse.ly history (circa 2012) was that “fancy” analytics is not as in-demand in the marketplace as “simple and easy analytics that actually work.”
Working closely with media companies, we began to learn more about their specific challenges and found opportunities to solve key problems. One emerging, but dominant issue was analytics. Analytics had the power to change the way media companies operated from the bottom up, yet the tools on the market were not built for content and were cumbersome to use.
We eventually chose to focus on the latter because, it turns out, users were very frustrated with the state of web analytics tools even for answering basic questions; we wanted to offer a simple, fast, and elegant solution to that well-defined problem.
We also decided that “human-machine hybrid” predictive power (aided by data visualization) is powerful and might be the best option in the short- and medium-term. This is, in many ways, the same conclusion that Wall Street came to about trading.
Check out this Medium post in which I draw parallels between the predictive analytics models developed for financial markets and the media industry.
The Future of Predictive Analytics
It’s only in very recent years that fully automated trading systems have started to take shape; instead, a lot of trading is done by high productivity individuals aided by things like a Bloomberg terminal. In content, there are some similarities. Analytics can’t replacement judgment, but it can augment decision-making. People who ignore analytics are “flying blind” versus competitors.
So what is the future of predictive analytics? “Anomaly detection” is an interesting development in the field. VividCortex, is one example of a company that has been working on the problem deeply in the server/database monitoring space.
It seems like anomaly detection is a tractable problem that can be solved by clever software, and it could yield actionable results for end users of analytics products. This may be more common in analytics tools as a way of “highlighting the out-of-the-ordinary” and helping users spot trends in real-time.
In your opinion, what is the difference between predictive analytics and “regular” analytics? How can you use one to influence the other? Chat with me on Twitter @amontalenti.